The current installment of our series [here] on growing the marketing function in scaling B2C businesses focuses on the interplay between the different marketing channels. More specifically, we are taking a closer look at how to account for the impact that these channels have on each other. Attribution models essentially apportion the benefit of a customer transaction to each channel.
Consider, the following customer journey which happens regularly at our portfolio company, azimo, a leading digital money transfer company: an immigrant, for instance from Poland, wants to send some money to family members.
He first types “Sending money to Poland” into Google and encounters the paid advert from azimo amongst others. He then goes to various money transfer websites where he may or may not register his details. At this stage he is just exploring and not (yet) sending any money. And over the next few days he both sees azimo’s retargeting creatives and receives emails from the company. Towards the end of the month he spots a TV advert from azimo and after reading some customer reviews decides that azimo has the best suited service for him, downloads the app and sends money to his eagerly waiting family members.
How then do you account for the impact that the various marketing channels have had on convincing this customer? Historically, all benefit was attributed to either the first or the last click.
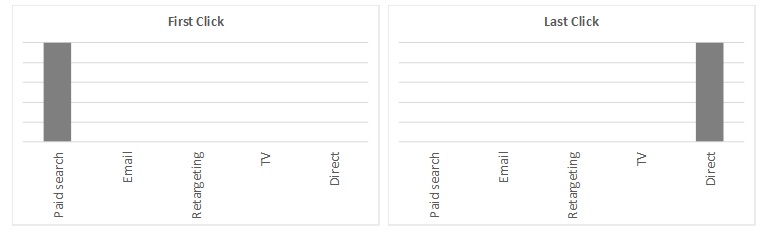
These models are obviously over-simplistic and diminish the positive effects the other interactions have had. One could then also consider attributing the benefit equally to the first and last touchpoints or weigh uniformly across all channels. These models approach reality a bit more, but are still generic.
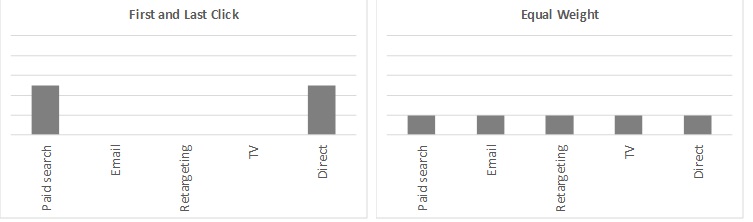
You could refine this analysis further by assigning individual weights to each step. By varying the inputs on the different steps you can test your assumptions and match your model with reality closer and closer.
However, the reality isn’t quite as easy. To test your model you need a significantly meaningful number of comparable customers. Think hundreds that should otherwise be comparable. In our azimo example this means the we should look only at Polish customers sending from the UK and first searching for a generic term online. This dependency on large numbers is precisely why attribution models usually become relevant in the scale-up phase that we at Frog focus on.
Even then, attribution models do not become a definitive science. On the one hand, the relative importance of marketing channels will likely evolve for you and therefore so will its impact. Further complications can lie in an omni-channel marketing mix, or more precisely in the offline channels, as it is not always possible to identify initial offline touchpoints if clients don’t identify themselves.
Therefore, attribution models deserve and require periodic revisions to enable the decision makers in the marketing functions of quickly scaling companies to make the best possible decisions.